Sitting in a UNC classroom, I was listening to a discussion about urban legends when one of my peers couldn’t help but link the concepts we were discussing in class (about what makes information and stories believable) to that of the Anti-Vaccine “Movement.” It was a soft comment, only lightly accusatory of the ignorance of anti-vax proponents. However, as she neared the end of her remark, another hand in the back row shot up.
I have a son with autism and sometimes people will come up to me and accuse me of making his brain that way because I vaccinated him. Even random people in the grocery store. It always makes me feel as if they’re implying that they’d rather my son be dead because of some horrible disease than be autistic. It makes me want to scream at them because the whole thing was started by some bogus science, that by now has been discredited ten-fold. My son’s brain is different, but he is alive and healthy.
The above testimony is paraphrased, but not exaggerated in its content and emotion.
We’ve all heard stories of people who believe vaccines cause autism or those about people who refuse to believe the clear connections between the two or that vaccines can cause permanent injuries. But those are just stories. In an effort to find out what real people were saying about vaccines and how and why they were saying it, I did what any logical college student would do.
I got on Twitter.
A Brief History of the Vaccine Debate:
In 1998, a British physician named Andrew Wakefield published a paper that linked the measles, mumps, and rubella (MMR) vaccine and autism in a peer-review journal known as The Lancet. After other researchers failed to replicate Wakefield’s findings and some questionable financial motives on the part of Wakefield were discovered, his work was discredited and most of his co-authors withdrew their support of the study and its conclusions. Wakefield has since been entirely discredited and the British Medical Journal even described his work as an “elaborate fraud.” However, 21 years later, Wakefield’s connection is still frightening people all over the world and has now led to one of the biggest global health crises to date.
For more information, visit this Wikipedia page and this incredibly brief and straight forward government website.
For further information, no, Andrew Wakefield does not have a Twitter account.
Data Collection Process:
In order to understand how people were discussing the issue of vaccines on Twitter, I kept a running archive of tweets that used the hashtag #Vaccines. Although simple, the direct wording helps prevent a thread of one-sided tweets from entering the archive (e.g. with hashtags like #VaccinesWork or #VaccineInjury). Using a Google Sheet template known as TAGS v6.1.9.1, I gathered tweets every hour for 6 days. However, when beginning my coding process, I realized analyzing thousands of tweets wasn’t practical. I first filtered out Retweets in order to avoid repetition in the data. Next, I took a random sample of 106 tweets and coded them for the following categories: Stance (opposed, neutral, support), Action Verbs (fearmongering, endorsing, reporting, refuting, questioning), Evidence for Stance (personal, big pharma, international, "fake news," science, general health), Gender (man, woman, n/a), Perceived Political Affiliation (1-5, 1 being most conservative, 5 being most liberal, 3 being neutral), and Media (link, picture, thread, none). It is important to note here that there was a reasonable amount of subjectivity in my coding. For example, perceived political affiliation was based on the user’s self-identification as often as possible, but for reporting news accounts and non-identifying users whose tweets were neutral or only slightly skewed, a political affiliation number of 3 was given. After coding all 106 tweets, data were collected into charts and graphs in order to form conclusions and gather insight into this general group of hashtag users.
Analysis:
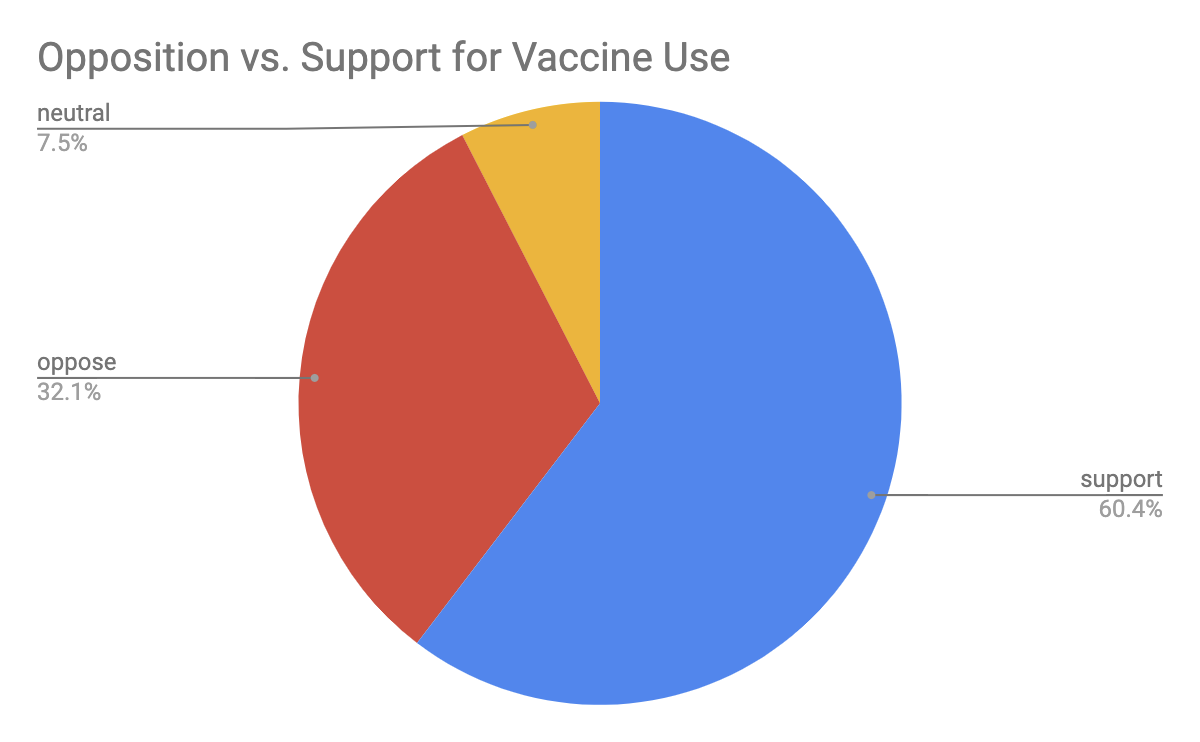
More than half of the tweets were in support of vaccines, and a surprising few were neutral. With how many news (and self-proclaimed news) accounts were tweeting, I had assumed that more tweets would be neutral. But, as with most things, biases can’t help but come through. Credible news accounts tended to be in support, while self-proclaimed news accounts were more likely to oppose vaccines.
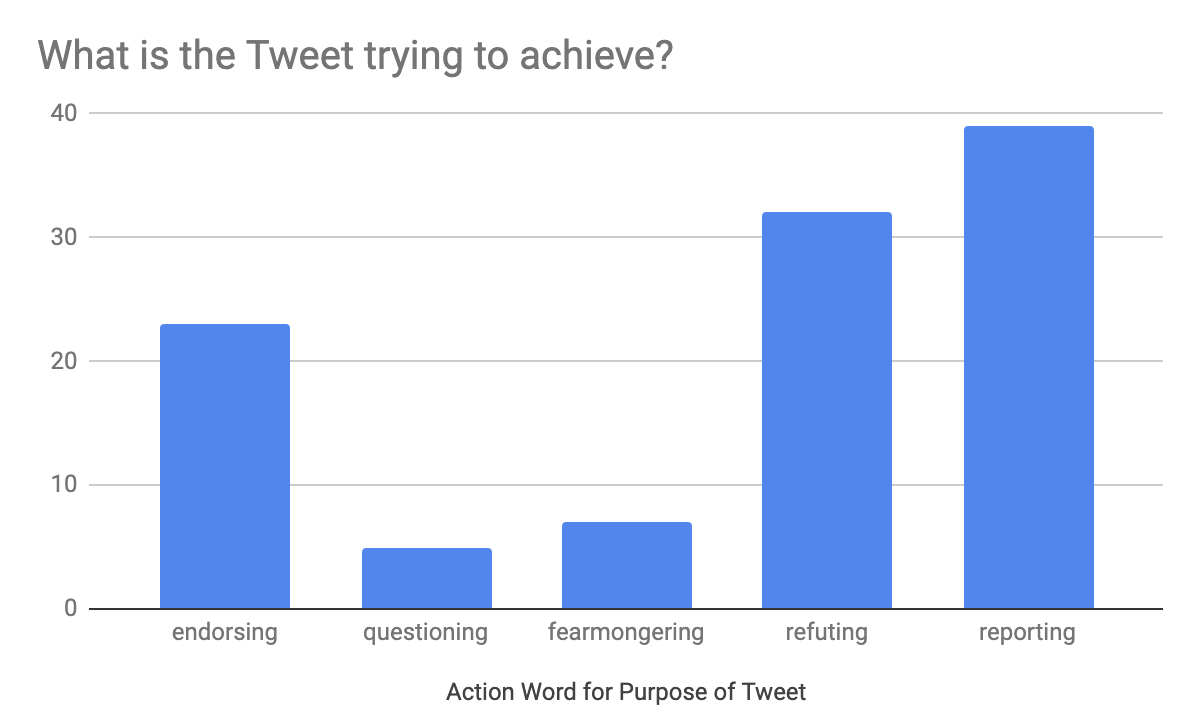
In analyzing the tweet’s function, I based my coding on why I presumed the tweet was sent in the first place, regardless of support or opposition to vaccines. More than a third of the tweets were “reporting” in some way. This included news sites primarily but also included users who were trying to support their own views, usually by adding website links. Refuting tweets were the next highest category of function, and those tweets were often responding to another person’s specific tweet or were intending to refute a general claim made by the respective “other side.” Endorsing tweets constituted around a fifth of the total sample, most of which were tweeted in support of vaccines with some kind of evidence to back up the safety and reliability of vaccines. However, a few tweets in this category were endorsing anti-vaccine ideas, though they too included evidence like vaccine injury stories. Few tweets were attempting to use people’s fear for persuasion. I was somewhat surprised by this, but also glad that most people stayed away from such tactics. Lastly, questioning tweets were the smallest coded group. These tweets were genuinely asking questions either about the vaccines as a whole or about why one side felt the way they did. Questioning tweets tended as well to be neutral.
Teen Girl Loses Memory of 3 Months of her Life and 1/3 of Her Body Weight after HPV Vaccination – Barely Escapes Medical Kidnapping
— Health Impact News (@HealthyNews2day) August 30, 2019
Read more>> https://t.co/MkTjQpxfIk #HPV #Vaccines #VaccinesInjure #BigPharma pic.twitter.com/dlEKBTr4VY
Example tweet for fearmongering. The connotations of words like "[memory loss]" and "kidnapping" intend to spark concern for the safety of vaccines.
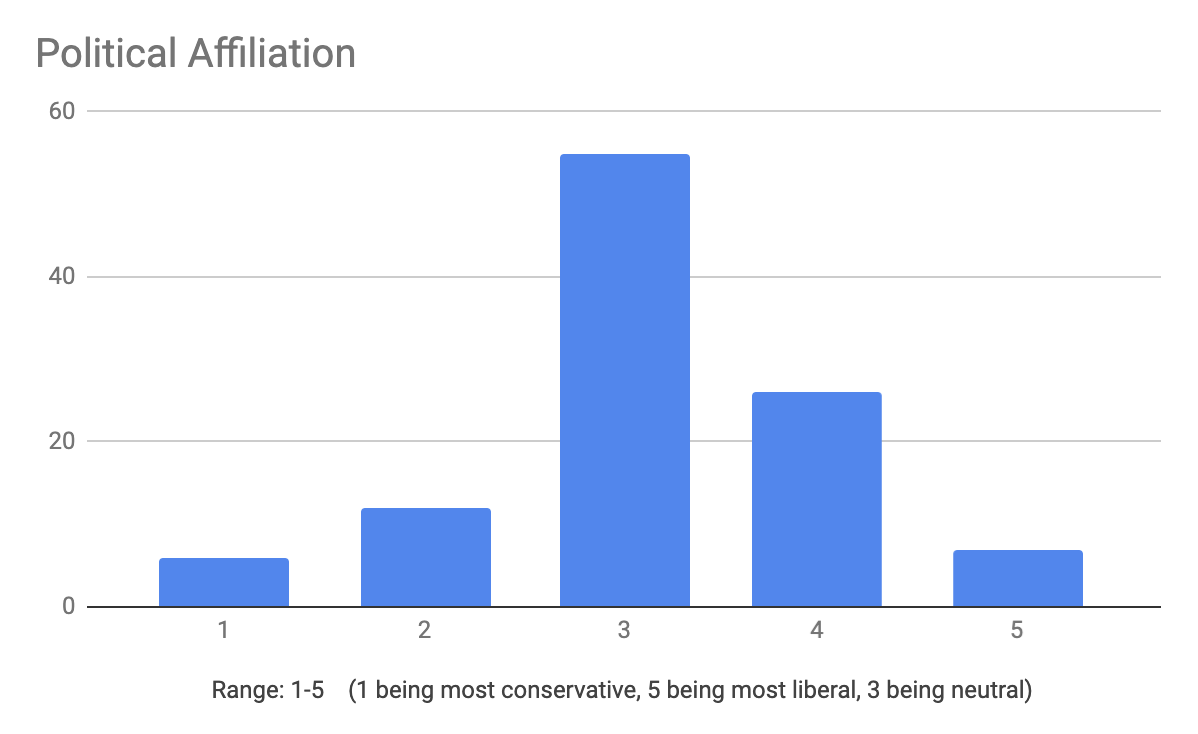
Political affiliation was difficult to assess, but I knew that it was going to be an interesting code to discover in its ability to show trends. A small disclaimer here is that 3 (neutral) is partially skewed because if a tweet did not take a direct political stance or it was a news account that was simply reporting, I recorded the affiliation as neutral. The one and five categories were assigned to individuals who had strong identifiers in their account bio; this includes profile pictures with MAGA hats, #BernieOrBust comments, etc. Two and four represent the tweets that showed only a small sway in one direction or the other. As a whole, there were slightly more liberal-leaning individuals tweeting about vaccines than any group besides neutral. Since this group is more likely to support vaccine use and more than half of the sample tweets were supporting, this finding does not come as too much of a surprise.
Did you ever have one of those days?
— Covfefe in Atlanta (@WatcherOnAWall) August 30, 2019
Is this the “Information Age” or the “Misinformation Age?"
Sometimes I feel like I’m Surrounded by Idiots.#Atheism#Science#Evolution#Vaccines#FlatEarth#Trump#WorstPresidentEver pic.twitter.com/IbgNXW3xYS
Example tweet for political affiliation. This tweet was coded as a "5".
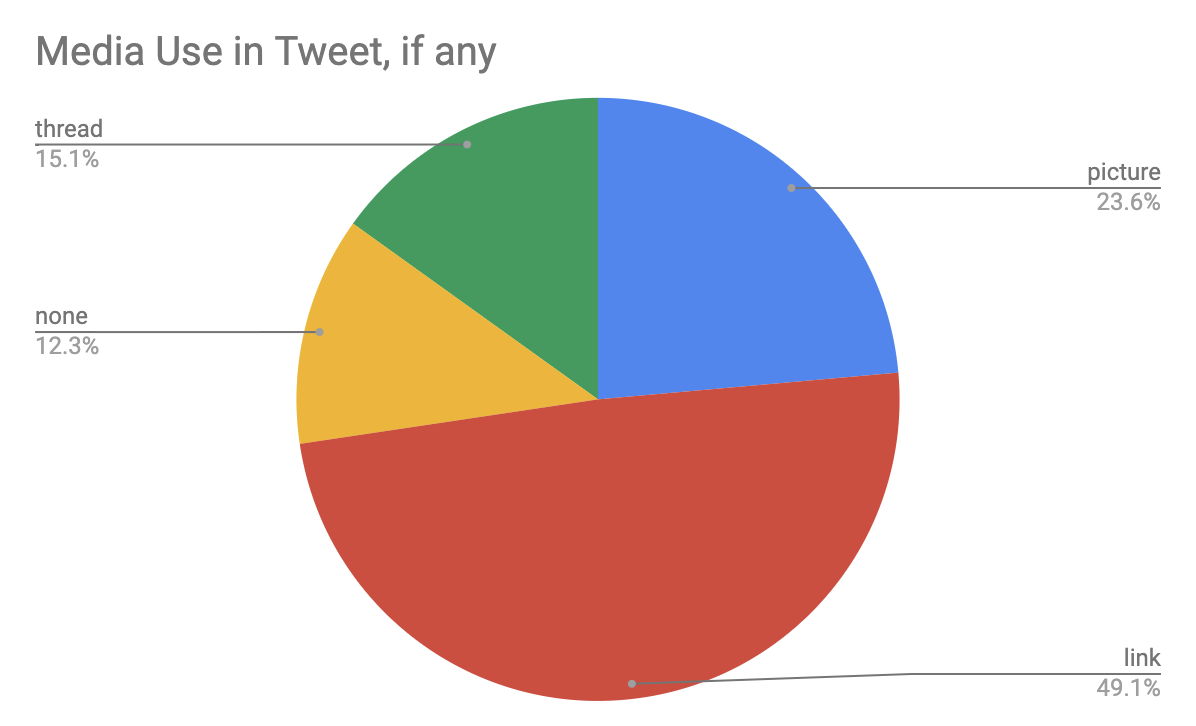
I was surprised by the findings of the Media coding. Very few tweets relied on the user’s words alone. Nearly three-fourths of the tweets contained supporting evidence like a link or picture. Some of the links were even advertising interactive quizzes where adults could discover which vaccines they were still in need of or for which they now needed the booster vaccine. Threads were defined as either a response tweet or a subtweet that included, in some fashion, the ability to read the preceding thread. I included this as media because usually, the threads bolstered or outright opposed the analyzing tweet’s own stance and often added to conversation and understanding as a whole. Personally, this ended up being the most interesting coding category.
There’s a vaccine for that. In the 21st century, no one should be seriously ill or dead from measles. Measles causes much more morbidity and mortality than the vaccine to prevent it does. #measles #vaccines https://t.co/ygSOocsKla
— Maureen Miller (@MMEPINYC) August 29, 2019
Example tweet for media use. A witty tweet and a link to a credible source were all it took for this tweeter to get their message across.
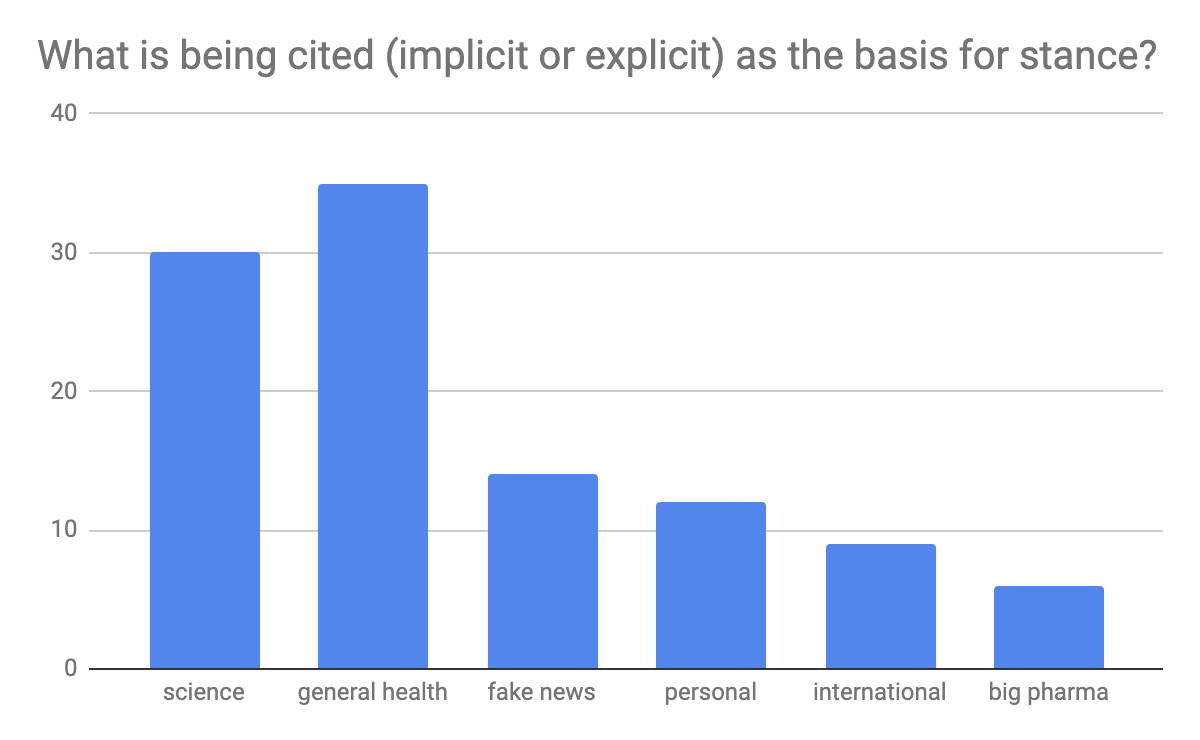
Lastly, I analyzed the evidence that people seemed to be concerned with the most and what seemed to influence the stance of a specific tweet. A significant majority of the sample tweets were appealing to the general health of the public or were relying on the credibility of the science behind their view. Both sides employed these methods rather evenly, though credible news accounts tended to rely heavily on verified science. Fake News evidence was also used by both sides, with supporters saying that anti-vax science is unfounded and false and opposers saying that pro-vaccine propaganda was falsely advertising a dangerous medicinal practice. Opposers would sometimes allude to big pharma capitalism as another reason to stay away from vaccines since they were supposedly only designed to put more money into the industry’s pocket. The international and personal codes were given to tweets that mentioned international public health or which used personal examples (both negative and positive, sometimes also international). This was also a fascinating category to analyze for me, as finding out the basis of someone’s argument is something I like to do in my everyday discussions. I find that seeking out a person's justifications for their opinions can be helpful in identifying the purposes and influences that they find to be most pertinent and valuable. However, in person, follow up questions are simple to construct and receive relatively quick responses. Attempting to identify someone’s reasoning is more difficult on the internet because direct, in-depth discourse is almost nonexistent on social media, and basically prohibited by Twitter's character limit. Nonetheless, it is still an important aspect of internet argumentation.
Conclusion:
I found my research to be illustrative of the general diversity of the vaccine dilemma. A majority of people are frightened, usually for their own safety, about the validity of vaccine use or the danger anti-vax mindsets can present to public health. Conducting research as I have done here can aid in the understanding of both sides and could lead to a time when some sort of conclusive stance is taken. As a whole, this Twitter “community” is struggling with the health crisis at hand, and many tweets do their best to be persuasive in the little space that they have. This also presents an element of evidence-giving that is unique to internet arguments in that they can provide links, pictures, gifs, quizzes, threads, etc. with the ease of a simple tap to their readers/listeners. However, the leading question still remains: how do we determine if those abundant bits of evidence are actually credible?
Disclaimers:
I personally support the use and safety of vaccines, therefore, some biases may appear in my analysis. I attempted to be as neutral as possible in an effort to protect the integrity of my research. Additionally, these findings are based on a very small sample size and may not consistently apply to the population as a whole. Alas, I am but one person, and doing a true sample of thousands of tweets would have been near impossible in the time given. I enjoyed this process and would encourage any person interested in analyzing Twitter arguments in such a way to utilize some of the methods I have employed here.